Thrashing feet, gliding swan – metadata challenges for environmental data science.
Tools for transparent data and model documentation (such as agreed metadata standards, controlled vocabularies and consistent quality representations) are important underpinning elements of environmental data science infrastructure. FAIR* resources can theoretically support robust and defensible data science, by supporting the discovery, fitness-for-use assessment, appropriate use / re-use, and clear provenance tracking of data and models.
However, such tidy curation is ever more challenging in a world with rapidly-expanding opportunities for interdisciplinary analysis of heterogeneous data, including commercial, volunteered and other emerging sources.
*Findable, Accessible, Interoperable and Reproducible
Getting to Net Zero
How data science at Cambridge University and Cambridge Zero’s engagement and research support work are driving technological and social change.
Data and data science for predicting the future of forests
Forests are cruicial to the global carbon cycle, harbour the majority of terrestiral biodiversity, and support the livelihoods of hundreds of millions of people around the world. But forests are changing with climate change, threatening their future and the future of all the services they provide. The future of a forest relies on the survival and success of individual trees within them, and while individual-based ecological models can reproduce observed long-term dynamics, the data needed to constrain them is missing for many parts of the world. In this talk I will discuss what data we have, and what is missing, to help us predict the future of forests. I will present new ideas about how cutting edge remote sensing, when analysed with modern data science approaches, can help us to understand forests at larger scales and finer details than ever before.
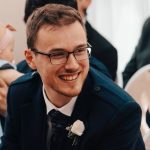
Kevin Donkers, Met Office
Skilful precipitation nowcasting using deep generative models of Met Office radar data
Precipitation nowcasting, the high-resolution forecasting of precipitation up to two hours ahead, supports the real-world socioeconomic needs of many sectors reliant on weather-dependent decision-making, particularly with the increasing likelihood of extreme events under climate change. State-of-the-art operational nowcasting methods typically advect precipitation fields with radar-based wind estimates, and struggle to capture important non-linear events such as convective initiations. Existing deep learning methods use radar data to directly predict future rain rates, without the need for dynamical modelling. While they accurately predict low-intensity rainfall, their operational utility is limited because their lack of constraints produces blurry nowcasts at longer lead times, yielding poor performance on rarer medium-to-heavy rain events. Here we present a deep generative model for the probabilistic nowcasting of precipitation from radar that addresses these challenges. Using statistical, economic and cognitive measures, we show that our method provides improved forecast quality, forecast consistency and forecast value. Our model produces realistic and spatiotemporally consistent predictions over regions up to 1,536 km × 1,280 km and with lead times from 5–90 min ahead. Using a systematic human evaluation by more than 50 expert meteorologists, we show that our generative model ranked first for its accuracy and usefulness in 89% of cases against two alternative methods. When verified quantitatively, these nowcasts are skillful without resorting to blurring. We show that generative nowcasting can provide probabilistic predictions that improve forecast value and support operational utility, and at resolutions and lead times where alternative methods struggle.