In this post, Dr Rob Smail (our recent AHRC Creative Economy Engagement Fellow) reflects on his research in using Machine Learning to explore Ruskin’s manuscripts.
What can computer Machine Learning reveal about Ruskin? During my time at The Ruskin as an AHRC Creative Economy Engagement Fellow, I’ve been exploring how the digitisation of The Ruskin Whitehouse Collection can create opportunities for new kinds of research.
The Ruskin Whitehouse Collection is the largest assemblage of Ruskin material in the world, and the most representative of Ruskin’s working practices across a diverse range of media. In addition to 7,400 letters and 29 volumes of manuscript diaries, it includes thousands of drawings, paintings and photographs – digitising all this material will take years. However, supported by the North West Consortium Doctoral Training Partnership (NWCDTP) and the Friends of the National Libraries (FNL), I’ve been able to work with the team at The Ruskin on a study to guide this work.
Our aims in this study were twofold. We wanted to set some basic digitisation standards and we wanted to experiment with using Machine Learning to trace connections across the full range of Ruskin’s works.
The Source Set
Our first task was to select a source set, with a manageable number of items to develop and refine our approach. Building on my previous work at the Lancaster Environment Centre, which focused on the historic flora of the Lake District, I decided to choose a source set that revealed Ruskin’s thoughts about the region.
Ruskin first visited the Lakes when he was 5, and he returned throughout his life before deciding to settle there in 1871, when he bought Brantwood, near Coniston. The last tour he made before buying Brantwood took place between late June and August 1867. On that occasion, Ruskin had come to the Lakes to recover from fatigue. His stay that summer helped him recoup, which is part of the reason he later made the region his home.
Surprisingly, Ruskin’s 1867 visit has received less attention than his other Lake District holidays. Therefore, we decided to centre our study in the letters he wrote during his tour, which had the added benefit of potentially enabling us to determine what it was about the Lakes that helped lift Ruskin’s spirits.
In all, we identified 53 letters. These included letters sent by the writer, Thomas Carlyle; the philologist, Fredrick Furnivall (of OED fame); the engraver, George Allen (who would later become Ruskin’s publisher) and the painter, Thomas Richmond. But the majority of the letters – 39 of the 53 – were sent to Ruskin’s cousin, Joan Severn, and his mother, Margaret.
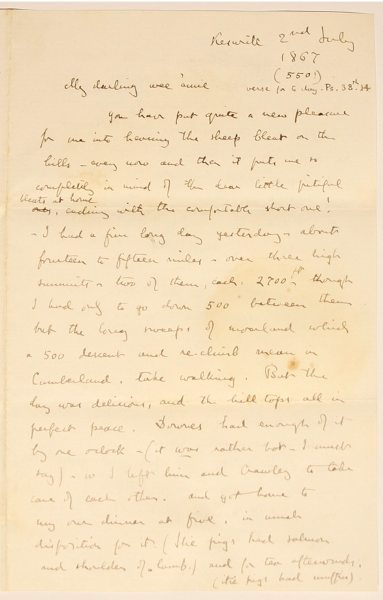
Digitising the Letters
Digitising these letters was a two-part process, which was supported by the contributions of two digitisation assistants: Claire McGann and Ben Wills-Eve. Working together, we created an accurate and faithful transcription of the contents of each letter, and then we encoded information about each letter’s structure and layout into each transcription.
After consulting current standards, we decided to adapt the ‘modest approach’ to XML (eXtensible Markup Language) encoding recommended by our colleague Andrew Hardie. Andrew’s approach provides a flexible way of using XML tag elements to encode extra information about the plain text transcriptions, whilst keeping the amount of tags added to a minimum. These elements, which appear inside chevrons, help capture different levels of semantic meaning, and they can help us ensure that information regarding each letter’s structure and layout is retained during the process of digitisation. In order to ensure that our approach was in keeping with best practices in the field, we built on Andrew’s model by selecting tag elements based on the standards of the Text Encoding Initiative (TEI).
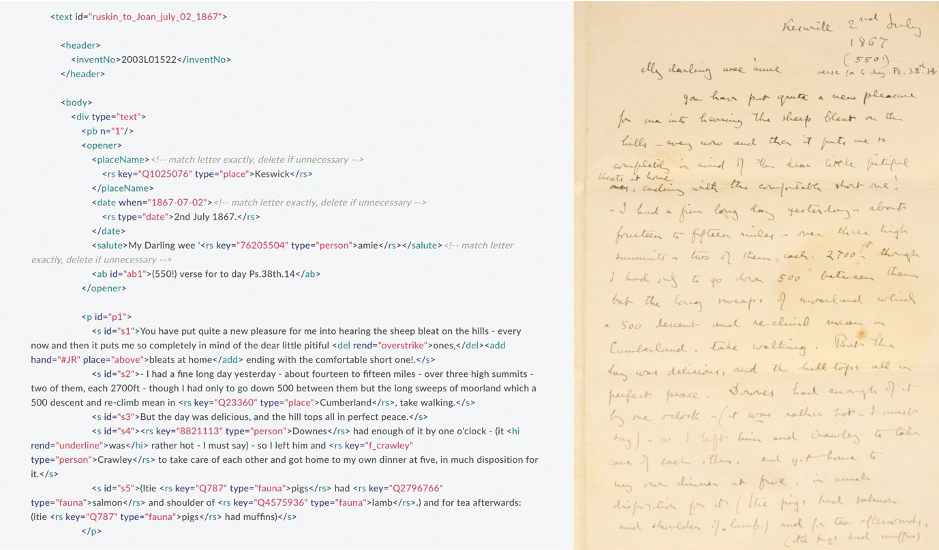
Using Machine Learning
Once we finished digitising all 53 letters in the source set, we were able to run a series of tests using Machine Learning approaches to examine them. One aspect of the letters we were keen to examine was whether we could use ‘classifiers’ to detect differences in the way Ruskin wrote to different correspondents.
Classifiers are algorithms that assist with predicative modelling. They’re often used in supervised Machine Learning research, where raw input data needs to be sorted on the basis of specific characteristics.
In this case, we used a classifier known as Naïve Bayes, which is based on Bayes’s Theorem and which has been shown to be reliable in the classification of texts. This theorem, formulated by the 18th-century minister and statistician, Thomas Bayes, helps calculate the likelihood of an event on the basis of characteristics that might relate to that event.
We were curious to see whether we could use Naïve Bayes to group the letters in the source set by recipient based on each letter’s stylistic characteristics.
Naïve Bayes works best when the algorithm can cross-reference several examples of the characteristics related to each classification. This process, which is sometimes called ‘training’, allows the classifier to learn which characteristics to associate with each group. So, we decided to restrict our experiment to the 39 letters in the source set to Ruskin’s mother, Margaret, and his cousin, Joan.
This gave us a small but sufficient sample with two clearly defined classifications: letters to Margaret and letters to Joan. Our aim was to determine if Naïve Bayes could correctly identify which letters were written to whom based on the words Ruskin used.
We split the letters in to two sets: a training set of 38 letters to which the recipient was known and a testing set of 1 letter, from which we’d removed the recipient’s name. Whereas the former was used to train Naïve Bayes; we used the latter to test whether the trained classifier was able to determine to whom the anonymised letter was sent.
We repeated the test 39 times, splitting the letters in every possible combination and then taking an average of all 39 predictions. We were pleased to find that Naïve Bayes was able to predict the recipient of the testing set correctly 87.2 percent of the time.
Our Findings
Our study confirms that there’s a discernible difference between the way Ruskin wrote to his mother and his cousin. Now, on the face of it, that might not seem all that surprising. Most of us adjust our style to suit our addressee.
What matters though, is that our findings demonstrate that – even with a modest source set – we can begin to train software to detect these differences and this can help us identify patterns in Ruskin’s writings across the whole collection.
Identifying these sorts of patterns gives us a new way of assessing Ruskin’s writing in different contexts over the course of his life, and an approach to determining when undated material was written and the identity of un-named correspondents. In future, it will be possible to train the software we’ve used with increasing accuracy and to extend it to different types of textual material, including Ruskin’s diaries.
These possibilities are exciting. They will allow us to reveal new links across the collection, providing researchers and visitors with deeper insights into both Ruskin’s works and his world.
__________
Dr Rob Smail received his PhD in History from the University of Manchester in 2012, and he completed his AHRC CEEF Fellowship at The Ruskin in 2019. His exploratory research with the Whitehouse Collection helped pave the way for further projects, including Digitising the Manuscript Letters of John Ruskin and Enriching understanding of natural-cultural heritage in the English Lake District.