Here we present a summary of scientific papers and other research outputs from the H-Unique project. Full papers can be downloaded in pdf format. Please not that these papers are technical papers intended for a specialist audience.
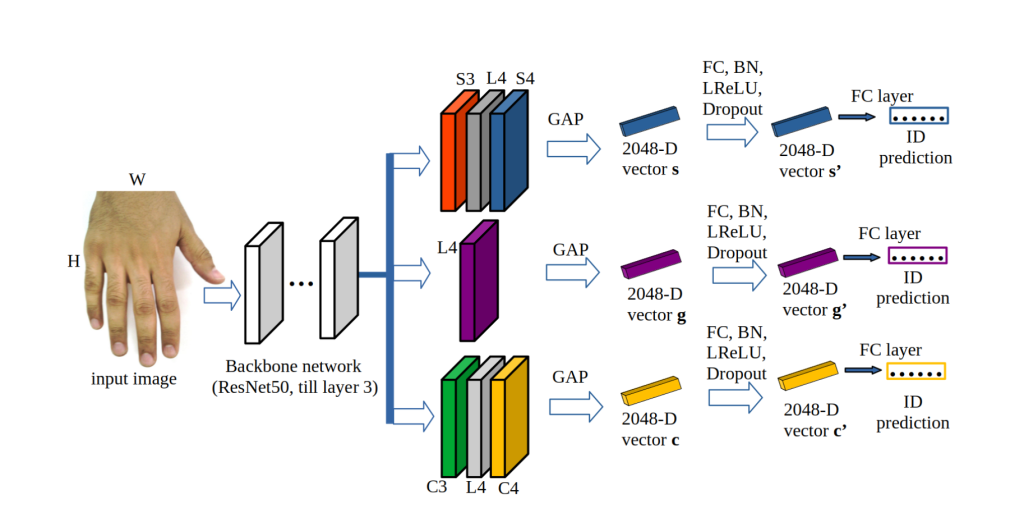
Multi-Branch With Attention Network For Hand-Based Person Recognition
Nathanael L. Baisa, Bryan Williams, Hossein Rahmani, Plamen Angelov, Sue Black
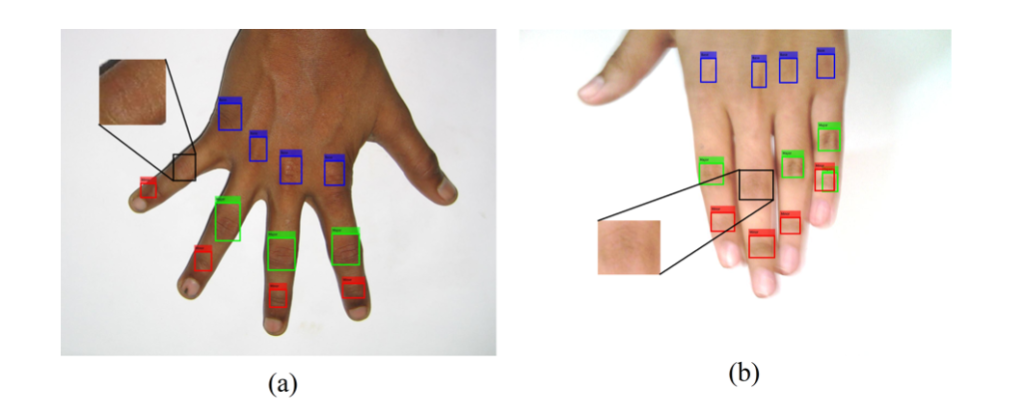
Robust End-to-End Hand Identification via Holistic Multi-Unit Knuckle Recognition
Ritesh Vyas, Hossein Rahmani, Ricki Boswell-Challand, Plamen Angelov, Sue Black, Bryan M. Williams
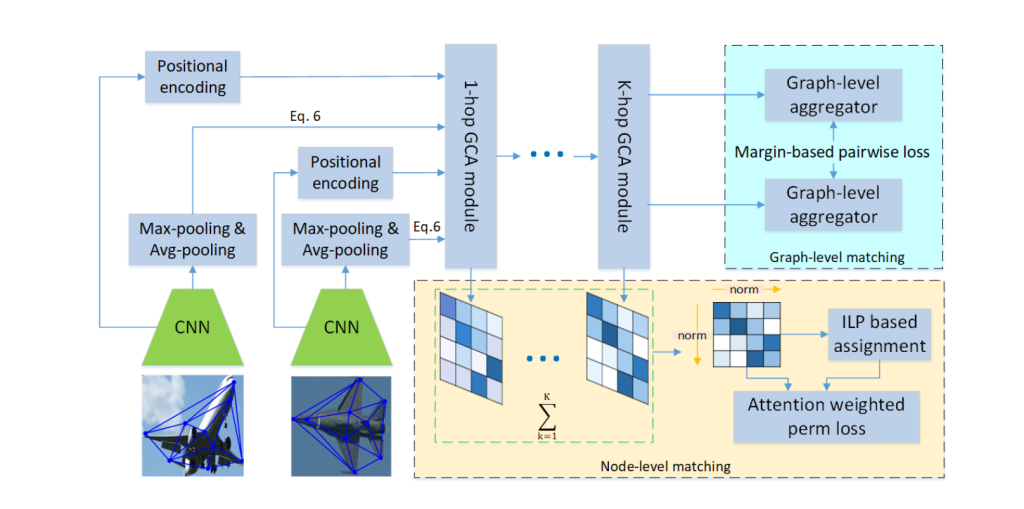
Graph-Context Attention Networks for Size-Varied Deep Graph Matching
Zheheng Jiang, Hossein Rahmani, Plamen Angelov, Sue Black, Bryan M. Williams
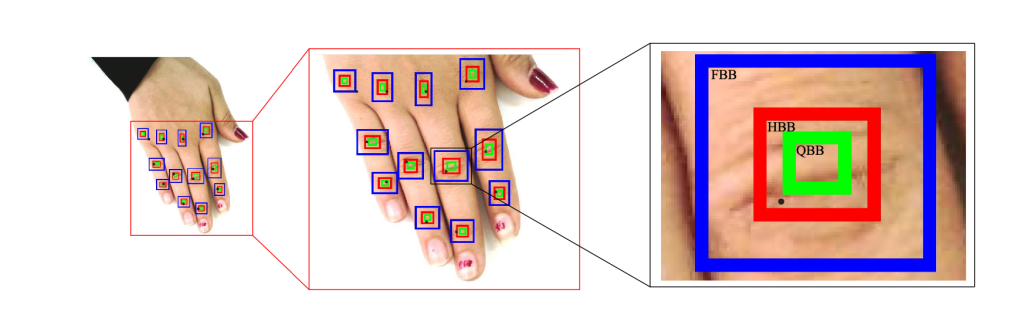
Ensemble-Based Bounding Box Regression for Enhanced Knuckle Localization
Ritesh Vyas, Bryan M. Williams, Hossein Rahmani, Ricki Boswell-Challand, Zheheng Jiang, Plamen Angelov, Sue Black
1. Multi-Branch With Attention Network For Hand Based Person Recognition
This paper describes an approach to identifying matching hands in a hand image dataset using an existing convolutional neural network (CNN) with additional attention network (an) layer intended to improve the discrimination of relevant areas of detail in hand images results in improved accuracy.
Citation: Baisa NL, Williams B, Rahmani H, Angelov P, Black S. Multi-Branch with Attention Network for Hand-Based Person Recognition. arXiv preprint arXiv:2108.02234. 2021 Aug 4.
2. Robust End-to-End Hand Identification via Holistic Multi-Unit Knuckle Recognition
This paper describes a novel approach to identification using knuckle crease patterns in hand images by identifying and comparing all visible knuckles (distal interphalangeal, proximal interphalangeal and metacarpopha-
langeal joints – referred to as major, minor and base knuckles) rather than just one knuckle.
Knuckle regions are first identified in the image using a trained region based CNN. After checking for quality of the extraction results, discerning features of each region are extracted and evaluated before using these to attempt to identify matching hand images. Evaluated on two large landmark datasets, the proposed framework achieves equal error rates (EER) of 1.0-1.9%, rank-1 accuracies of 99.3-100%
Alongside the paper, an online tool is also published, which allows the exploration of accuracy results obtained from various knuckle combinations.
Citation: Vyas R, Rahmani H, Boswell-Challand R, Angelov P, Black S, Williams BM. Robust end-to-end hand identification via holistic multi-unit knuckle recognition. In 2021 IEEE International Joint Conference on Biometrics (IJCB) 2021 Aug 4 (pp. 1-8). IEEE.
3. Hand-Based Person Identification Using Global and Part-Aware Deep Feature Representation Learning
This paper explores the use of a mixed global and part-aware CNN which works on both global and local image features for matching of hands in a dataset.
Citation: Baisa NL, Williams B, Rahmani H, Angelov P, Black S. Hand-based person identification using global and part-aware deep feature representation learning. In 2022 Eleventh International Conference on Image Processing Theory, Tools and Applications (IPTA) 2022 Apr 19 (pp. 1-6). IEEE.
4. Graph-Context Attention Networks for Size-Varied Deep Graph Matching
This paper describes a novel new approach to the identification and extraction of lineear features in images as graph of connected nodes. This has particular relevance for the mapping of hand veins, where the connections between vein segments are a key factor in identification.
Citation: Jiang Z, Rahmani H, Angelov P, Black S, Williams BM. Graph-Context Attention Networks for Size-Varied Deep Graph Matching. In Proceedings of the IEEE/CVF Conference on Computer Vision and Pattern Recognition 2022 (pp. 2343-2352).
5. Ensemble-Based Bounding Box Regression for Enhanced Knuckle Localization
In this paper we present an enhanced method for the robust and accurate location of knuckle regions in hand images using multiple region based CNNs (R-CNN) to identify knuckle regions and corrobarate each other’s results to provide superior localisation accuracy and classify the quality and usability of regions for biometric analysis
Citation: Vyas R, Williams BM, Rahmani H, Boswell-Challand R, Jiang Z, Angelov P, Black S. Ensemble-Based Bounding Box Regression for Enhanced Knuckle Localization. Sensors. 2022 Feb 17;22(4):1569.
6. A Probabilistic Attention Model with Occlusion-aware Texture Regression for 3D Hand Reconstruction from a Single RGB Image
Citation: Jiang, Zheheng, Hossein Rahmani, Sue Black, and Bryan M Williams. “A Probabilistic Attention Model with Occlusion-aware Texture Regression for 3D Hand Reconstruction from a Single RGB Image.” 2023.