To address the open challenge of provisioning self-organizing capabilities in future large-scale distributed power networks, such as the Smart Grid, we propose a paradigm shift to support real-time adaptivity.
Our objective is to enable real-time self-maintenance, self-healing, and self-reconfiguration capabilities for next-generation distributed power systems.
Our novel approach offers:
- A scalable, high-accuracy, real-time distributed monitoring scheme for highly dynamic and complex systems.
- An autonomous search and learning framework that balances exploration and exploitation through self-control.
- An autonomous network topology reconfiguration framework to adapt to dynamic changes in supply and load demands.
Our project is structured into three key phases, each addressing specific challenges:
Phase 1: Distributed Monitoring
Key challenges:
- Deriving an optimal set of subsystems for distributed monitoring with sufficient information to generate analytical hypotheses.
- Differentiating anomalies resulting from internal and external threats.
- Dealing with uncertainties in both endogenous and exogenous aspects.
|
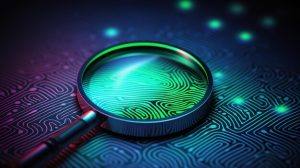 |
Phase 2: Autonomous Search and Learning
Key challenges:
- Characterising the operating environment of the complex system to satisfy the balance between exploration and exploitation.
- Managing the combinatorial explosion in machine learning search space for interdependent and growing power grids.
- Balancing the multiple power flow streams from renewable energy sources.
|
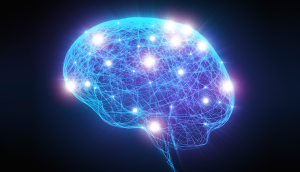 |
Phase 3: Network Topology Reconfiguration
Key challenges:
- Ascertaining where the learning agents offer the most benefit for online operation.
- Developing a learning-based understanding of autonomous network reconfiguration.
- Developing a highly automated testing framework for comprehensively evaluating algorithms and tools under dynamic operating scenarios.
|
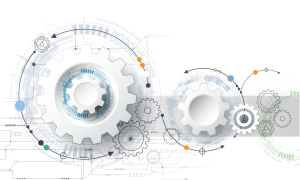 |
|
|
|
|